Thanks to publications from national registries or cohorts, most physicians can provide prognostic information at a population level. However, at an individual level, this information is more difficult to assess because of the multiplicity of risk factors. In the context of chronic diseases, synthetic prediction scores of clinical events have become increasingly popular. These scores may help patients and physicians in a shared decision-making and facilitate the implementation of the P4-medicine (predictive, personalized, preventive and participative) in clinical practice.
In the SPHERE unit, we developed and validated several prognostic scores in concrete clinical contexts:
- KTFS is a prognostic score of kidney recipient return-to-dialysis computed at 1-year post-transplantation
Link to KTFS Read the publication
- 1-RRS is a prognostic mortality scores in kidney transplant recipients calculated at 1-year post-transplantation
Link to 1-RRS Read the publication
- 1-RBI is a diagnostic score of abnormal histology on renal transplant biopsy at 1-year post-transplantation
Link to 1-RBI Read the publication
- SMILE is a clinical-based model for predicting the risk of residual disability at 6 months post-relapse in Multiple Sclerosis
Link to SMILE Read the publication
- DynPG is a dynamic predictive score of patient and graft failure risk that could be updated continuously during patient follow-up
Link to DynPG Read the publication
- DynHFH is a dynamic predictive score of heart failure hospitalization for diabetic patients
Link to DynHFH Read the publication
The SPHERE unit is involved in research about methodological issues surrounding prognostic modeling. Several methodological milestones were achieved on prognostic modeling as for instance:
- Le Borgne et al. proposed the weighted and standardized time-dependent ROC curve receiver operating characteristic curves to assess the intrinsic discriminative capacities of a prognostic marker in presence of confounding factors.
Read the publication
- Asar et al. considered robust joint modeling for longitudinal and survival data to improve accuracy of dynamic predictions in presence of longitudinal outliers.
Read the publication
- Fournier et al. proposed to assess the global predictive performance of dynamic predictions by using a R2-curve.
Read the publication
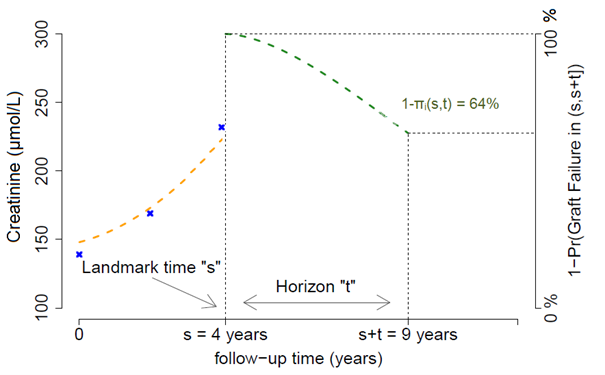
Causality
Prediction and causation have often been considered two different fields. Yet, the first step of causal modeling remains prediction. When using propensity score-based methods, this prediction step consists of predicting the exposure; when using a G-computation approach, it consists of predicting the outcome. Both approaches suppose using patients’ characteristics for these predictions. The SPHERE unit have initiated methodological researches on causal inference since several years.
- Lenain et al. used time-dependent propensity score to appraise the benefit of kidney transplantation compared to being in dialysis awaiting transplantation.
Read the publication
- Chatton et al. compared several approaches (g-computation, inverse probability of treatment weighting, full matching, targeted maximum likelihood estimator) aiming to control confounding bias in causal inference.
Read the publication
- Foucher et al. assessed the causal effect pre-emptive kidney transplantation from deceased donors from a cause-specific Cox models with propensity scores.
Read the publication
We follow our research on causality with new approaches coming from Machine Learning techniques when the exposure/treatment is time-dependent and for time-to-event outcomes.
We also plan to investigate methodological challenges regarding the study of causal relationships between numerous agents to model self-reported outcomes data or longitudinal data of psychiatric syndromes. Indeed, using networks analyses such as the paradigm of “network psychometrics” could help to unravel the interdependencies between the items of self-reported outcomes.
A study of the network of relationships between the items of a self-reported outcome measuring quality of life in chronic diseases is conducted. Using structural equation modeling, future works on studying the cross-lagged, mediation and remnant causal relationships on the longitudinal evolution of multiple psychiatric diseases are planned.